Project TuMM: Using a Tunable Knob for Reducing Makespan of MapReduce Jobs in a Hadoop Cluster.
The Problem:
In a classic Hadoop cluster, there are multiple task slots: map slots and reduce slots. Each job consists of multiple map and reduce tasks and each task slot can process only one task at any given time. The slot type indicates which type of tasks (map or reduce) it can serve. By default, Hadoop uses fixed numbers of map slots and reduce slots throughout the lifetime of a cluster. We argue that such static settings cannot yield the optimal performance in makespan for a batch of jobs with varying workloads.
Case 1: Better performance with more map slots than reduce slots
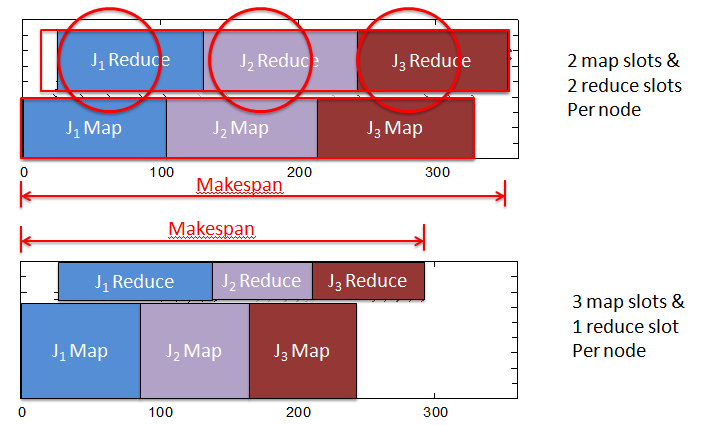
Case 2: Better performance with equal number of map slots and reduce slots
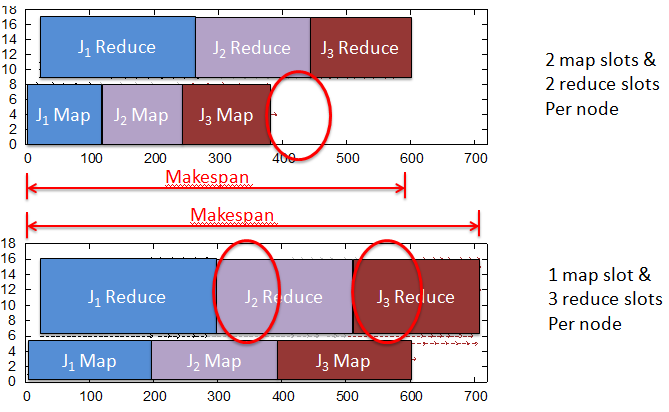
Our Solution:
Motivated by this problem, we propose a new approach, TuMM to improve the makespan performance for a batch of jobs under FIFO (First-In-First-Out) Scheduler. Using FIFO, there are at most two jobs running concurrently, job (i) in map phase and job (i-1) in reduce phase. The inappropriate setting of map/reduce slots may lead to extra overhead because of two cases: (a) the reduce slots will be idle if job (i)'s map phase is completed later than job (i-1)'s reduce phase; (b) the map slots will be idle if job (i)'s map phase is completed earlier than job (i-1)'s reduce phase. In this case, our goal is to achieve (c) by automating the slot assignment ratio between map and reduce tasks in a cluster as a tunnable knob for reducing the makespan of MapReduce jobs.
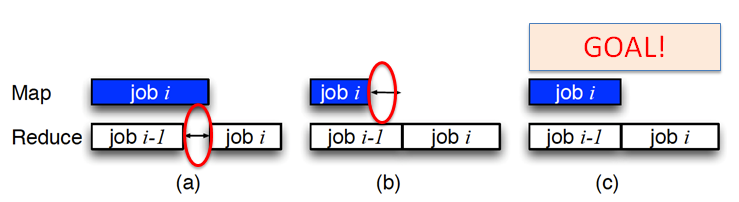
More specifically, the mechanism of TuMM is as follows:
- Keeps on updating the remaining workload (the remaining execution time with just one task slot) in map/reduce phase of each job based on the average execution time of one task and the number of remaining tasks.
- Adjust the slot ratio dynamically in the cluster to match the ratio of the remaining workload in map phase to the one in reduce phase. .
- Update the slot ratio based on feedback control to provide more map slots in the cluster since the execution time of one task will be extended when more map tasks are running concurrently.
The implementation of TuMM is based on Hadoop version: 0.20.2. Two modulers are created in JobTracker: Workload Monitor collects past workload information such as execution times of completed tasks and to estimate the workload of currently running map and reduce tasks; Slot Assigner adusts the ratio between map and reduce slots in the Hadoop cluster for each slave node. In addition, we modified the TaskTracker and JvmManager at each slave node to check the number of individual map and reduce tasks running on that node.
The Evaluation:
Experiment Settings:
-
Purdue MapReduce Benchmarks Suite
- Benchmarks: Inverted Index, Classification, Histogram Rating, Word Count, Grep
- Input files: 7GB wiki category links data, 10GB Netflix movie rating data
- Hadoop Cluster
- m1.xlarge Amazon EC2 instances
- 1 master node and 4 slave nodes
- 4 task slots on each slave node
Experiment Results:
- Homogeneous Workloads:
- Heterogeneous Workloads:
- Mix of five benchmarks
- Johnson's Algorithm is used to optimize the makespan of static slot configuration by adjusting the execution sequence of a batch of jobs.
- Execution Details with Heterogeneous Workloads:
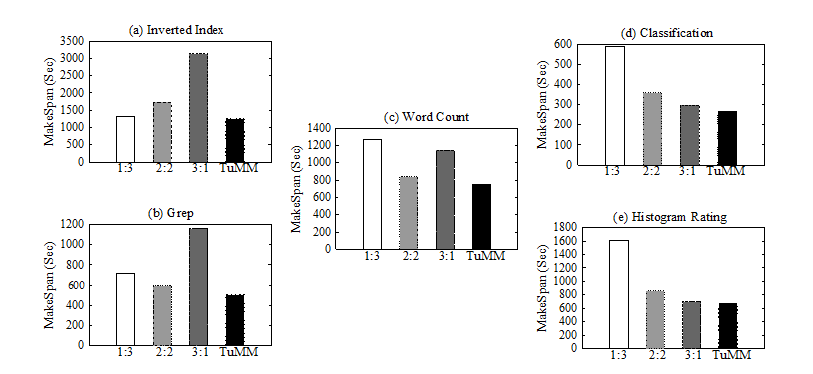
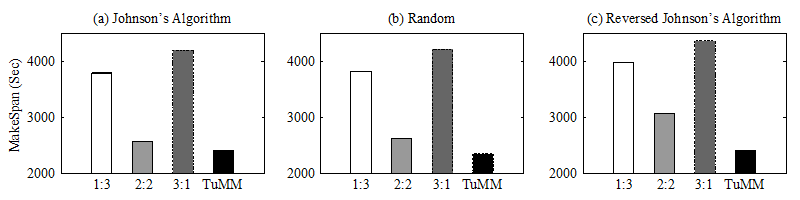
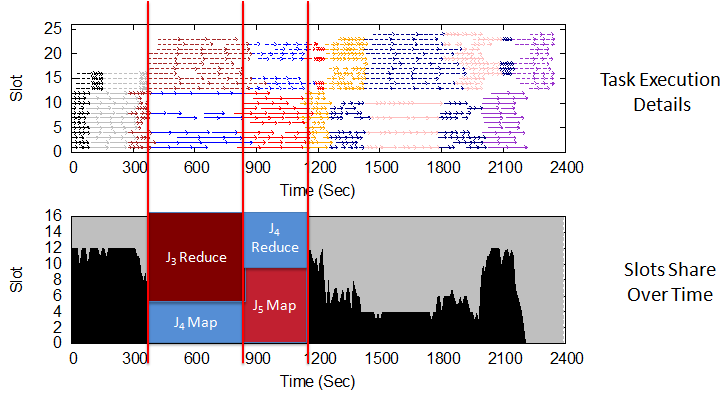
Publication :
FRESH: Fair and Efficient Slot Configuration and Scheduling for Hadoop Cluster
[CLOUD'13 ]The 6th IEEE International Conference on Cloud Computing, Santa Clara, CA, June 2013